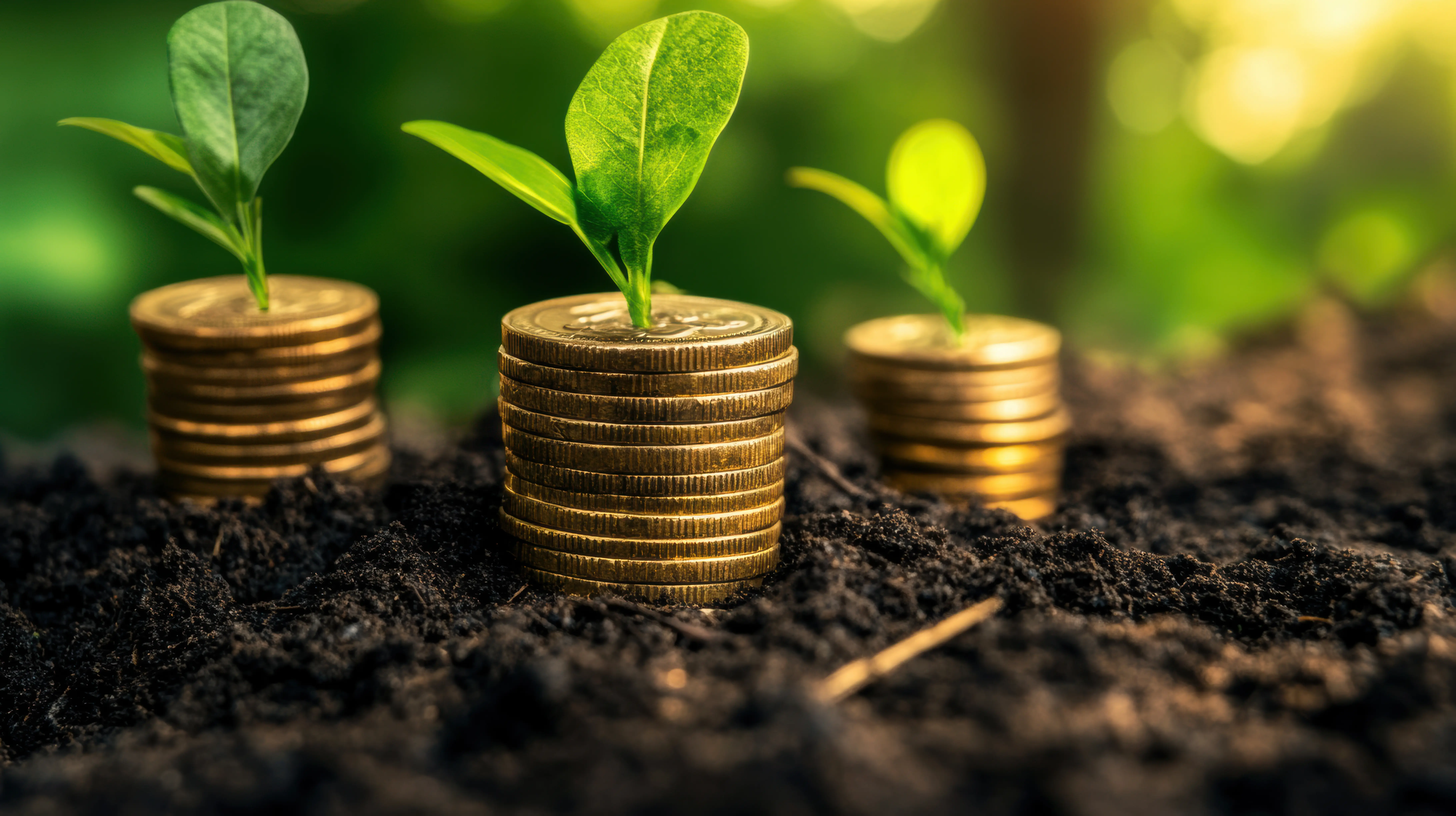
Funded Seed Projects
Accordion Content
The following interdisciplinary research projects received seed funding. These projects address challenges identified through Research Ideation Forums held by the Office of the Vice Provost for Research.
-
Principal Investigators
- Waheed U. Bajwa, Rutgers–New Brunswick School of Engineering; Electrical and Computer Engineering
- David Zald, Center for Advanced Human Brain Imaging Research, Robert Wood Johnson Medical School
- Linden Parkes, Center for Advanced Human Brain Imaging Research, Robert Wood Johnson Medical School
- Avram Holmes, Center for Advanced Human Brain Imaging Research, Robert Wood Johnson Medical School
-
Principal Investigators
- Adam Gormley, Rutgers–New Brunswick School of Engineering; Biomedical Engineering
- David Sleat, Center for Advanced Biotechnology and Medicine, Robert Wood Johnson Medical School
Co-Investigator
- Peter Lobel, Center for Advanced Biotechnology and Medicine, Robert Wood Johnson Medical School
-
Principal Investigators
- Mark van der Maas, Rutgers–New Brunswick School of Social Work, Center for Gambling Studies
- Jim Samuel, Rutgers–New Brunswick Edward J. Bloustein School of Planning and Public Policy; Informatics
-
Principal Investigators
- Ahmed Aziz Ezzat, Rutgers–New Brunswick School of Engineering; Industrial and Systems Engineering
- Josh Kohut, Rutgers–New Brunswick School of Environmental and Biological Sciences; Marine and Coastal Sciences
-
Principal Investigators
- Woojin Jung, Rutgers–New Brunswick School of Social Work, Global Health Institute
- Dimitris Metaxas, Rutgers–New Brunswick School of Arts and Sciences; Computer Science
- Dimitrios Ntarlagiannis, Rutgers–Newark School of Arts and Sciences; Earth and Environmental Sciences
- Min Xu, Rutgers–New Brunswick School of Arts and Sciences; Statistics
- Yuan Liao, Rutgers–New Brunswick School of Arts and Sciences; Economics
Co-Investigators
- Quentin Stoeffler, University of Bordeaux School of Economics
- Maryam Hosseini, Massachusetts Institute of Technology, City Form Lab
- Simone Nsutezo, Microsoft Research, AI for Good Lab
- Anthony Ortiz, Microsoft Research, AI for Good Lab
-
Principal Investigators
- Xiangmin (Helen) Liu, Rutgers–New Brunswick School of Management and Labor Relations, Human Resource Management, Labor Studies and Employment Relations
- Youngfeng Zhang, Rutgers–New Brunswick School of Arts and Sciences, Computer Science
Co-Investigators
- Adrienne Eaton, Rutgers–New Brunswick School of Management and Labor Relations, Labor Studies and Employment Relations
- Todd E. Vachon, Rutgers–New Brunswick School of Management and Labor Relations, Labor Studies and Employment Relations
Through recruitment and hiring processes, employers play a critical role in shaping economic opportunities for workers and their families by matching job candidates with vacancies. The integration of recommender systems in hiring practices has the potential to uncover the hidden workforce, reduce search costs for job seekers, and mitigate certain human biases. However, the pervasive use of data-driven recommender systems in hiring and recruitment has radically transformed the way we recognize, assess, and address fairness issues in employment decisions. In this project, our interdisciplinary team aims to develop a human-AI collaborative approach that seeks to enhance fairness throughout the entire lifecycle of job recommender systems, encompassing their development, adoption, and deployment.
-
Principal Investigators
- Meiyin Liu, Rutgers-New Brunswick School of Engineering, Department of Civil & Environmental Engineering
Co-Investigators
- Dake Zhang, Rutgers-New Brunswick Graduate School of Education, Department of Educational Psychology
Assessment biases widely exist in higher education and are understudied, especially for problem types that do not have standard answers and/or require multi-step reasoning, such as word problem-solving in college engineering disciplines. A common bias comes from human graders’ limited capacity to identify and understand students’ usual and low-frequent problem-solving strategies, which are more commonly observed in students with under-represented minority backgrounds. These unusual and low-frequent strategies represent students’ mathematical reasoning. However, they are often neglected by human graders, thus making evaluations biased against minority students.
To enhance Diversity, Equity, and Inclusion (DEI) in higher education engineering disciplines, this research proposes an automatic grading system for evaluating students’ word problems, particularly for detecting and understanding unusual and low-frequent problem-solving strategies. Additionally, instead of only assigning a score, this proposed project will contribute to the field by taking an initial effort to generate feedback with human-interpretable justifications. Specifically, this research will leverage visual representation of reasoning processes in learning sciences and graph technology in artificial intelligence. This research will develop a prototype web application hosted on Rutgers’ eLearning platform and adopt it in real engineering classes at Rutgers University.