Ryan Sills
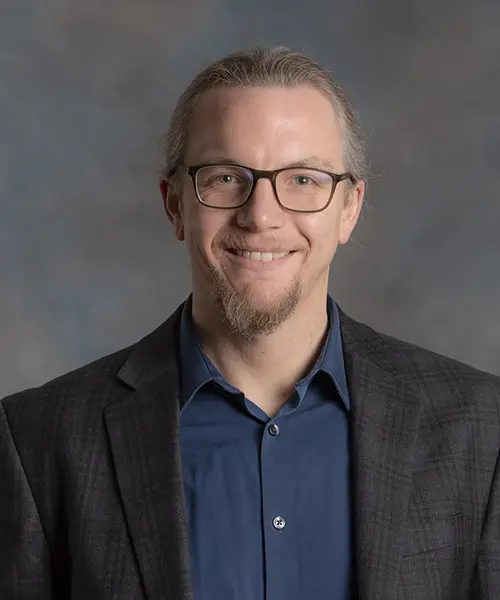
Biography
Prof. Sills’ work is focused on utilizing theory and simulations to better understand deformation and damage mechanisms in materials. Work in his group ranges from atomistic modeling where discrete atoms are represented up to continuum scale modeling used to simulate large engineering structures (e.g., vehicles, spacecraft). His research has three major thrusts: (1) construction of predictive, physics-based material models that can be used in engineering applications, (2) use of materials modeling to aid and inform development of advanced materials, and (3) development of open-source software tools that accelerate research. Prof. Sills is the Editor-in-Chief of the Journal of Materials Science: Materials Theory, a fully open-access journal published by Springer Nature.
In his group’s work, Prof. Sills has leveraged machine learning in several ways. With collaborator Prof. Aziz Ezzat of Industrial and Systems Engineering, his group developed a method for constructing coarse-grained constitutive laws using Gaussian process regression, a non-parametric machine learning technique. This method supplants ad hoc techniques commonly by the research community. Prof. Sills is also the inventor of the finite-element-based physics-informed neural network (FE-PINN) methodology, which efficiently trains neural network surrogate models using existing FE codes. Finally, Profs. Sills and Aziz Ezzat have developed a data-driven methodology for analyzing results from atomistic simulations.